CompressiveDistortion.3
From CNBH Acoustic Scale Wiki
|
Roy Patterson
CAR-FAC and the distortion observed in Patterson, Handel, Yost and Datta (1996)
This section is in the first stages of development.
It is typically not difficult to produce a computational explanation of the pitch of a periodic sound, including pulse-resonance sounds. They produce auditory figures with a vertical ridge and the time interval associated with the veritcal ridge provides a very good estimate of the period of the pitch that a listener will hear. It is more difficult, however, to explain the pitch strength or salience of quasi-periodic sounds and sounds where the temporal regularity is marginal, of which there are many. Thus, it is now common to test the accuracy of computational models of pitch with stimuli where the salience of the pitch can be varied from negligible to dominant.
One stimulus that is often used to manipulate pitch strength is iterated rippled noise (IRN), or Regular Interval Noise (RIN). It is generated, as indicated in Figure 1, by repeatedly delaying a block of white noise by a fixed amount and adding the delayed versions of the noise back to the original noise. This delay and add procedure has the effect of selectivlty increasing the proportion of the time intervals in the NAP of the sound at the interval of the delay. With one or two iterations, the pitch component of the perception is weak and the perception is dominated by the hiss of the noise used to generate the stimulus. But as the number of iterations increases, the pitch becomes stronger and the hiss becomes weaker, and between 8 and 16 iterations, the pitch comes to dominant the perception as the hiss dies away.

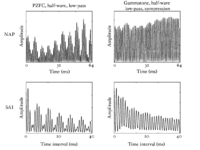
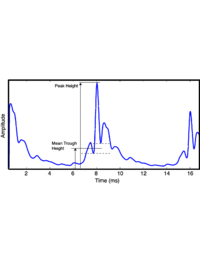
shows SAI temporal profiles generated with the dcGC, the PZFC and the gammatone filterbank with and without logarithmic compression for IRN stimuli. The profiles for the zero-iteration IRN (just noise) show little temporal structure, as we would expect. It is clear from inspection that the gammatone filterbank with logarithmic compression produces profiles in which the peak in the profile which corresponds to the perceived pitch is much less strong than for the other filterbanks. For comparison, the results from the gammatone filterbank without logarithmic compression are included. The pitch strength estimates are stronger than for the log-compressed gammatone, but the linear gammatone is not a physiologically reasonable auditory filter model as it has no compression, and it is included in these experiments only for comparison with the other compressive filterbanks.
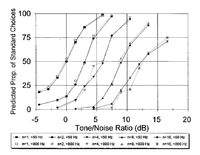
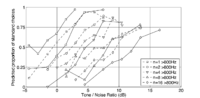
Chapter 5 of his dissertation (Section 4), Walters (2011) compares the periodicity peak produced by several time-domain models of auditory processing including the original version of the PZFC. A research collaboration with Nick Clark at IHR had led to the discovery that both the PZFC and the dcGC provide a more pronounced peak in the temporal profile of the auditory image than the standard gammatone filterbank The normalised pitch-strength measured for IRN stimuli was compared with the pitch strength measured for IRN in perceptual experiments. Patterson et al. (1996) performed a series of experiments on the human perception of IRN by comparing the pitch strength of IRN stimuli and tonal stimuli masked with noise (Yost, 1996; Yost et al., 1998; Patterson et al., 2000; Handel and Patterson, 2000). Subjects compared IRN with different numbers of iterations to a tonal stimulus (256-iteration IRN with a 16ms delay time) masked with noise. Subjects were asked to select the stimulus with the stronger pitch strength as the SNR of the noise-masked tonal stimulus was changed. In their experiments two conditions were tested, in which the stimuli were high-pass filtered with a cutoff frequency of either 50Hz or 800Hz. The 800Hz filter condition was designed to exclude the resolved harmonics from the stimulus. The pitch strength measure described above was used to model the data of Patterson et al. (1996), using the techniques described in that study. The original results from the perceptual experiment are plotted in Figure 4, the predictions made by Patterson and Yost's model are shown in figure Figure 5 and the predictions made using the current measure on an SAI made using a PZFC filterbank are plotted in Figure 6. In each case, the horizontal axis is the tone to noise ratio, and the vertical axis is the predicted proportion of the time that the standard noise-masked tonal stimulus was picked as having a higher pitch strength than the IRN stimulus. In practice the results of Patterson et al. (1996) did not show much difference between the 50Hz and 800Hz condition, and a similar result was seen when using the pitch strength measure described here, so only the more challenging 800Hz condition is compared.
It is clear from these results that the dcGC gives rise to a considerably stronger pitch feature in the temporal profile of an IRN stimulus than do the other filterbanks. While the pitch feature from the PZFC is stronger than that from the log(gammatone) filterbank, the results are on a par with the results from the linear gammatone. Standard deviations for the pitch strength measures are given in brackets after each value.
The results suggest that the processing performed by the dcGC is fundamentally different to that performed by the PZFC for these stimuli. In order to determine why performance with the PZFC is inferior to that with the dcGC, we now turn to a deterministic signal to perform several experiments on the AGC of the PZFC.
References
- Handel, S. and Patterson, R.D. (2000). “The perceptual tone/noise ratio of merged, iterated rippled noises with octave, harmonic, and nonharmonic delay ratios.” J. Acoust. Soc. Am., 108, p.692-695. [1]
- Ives, D.T. and Patterson, R.D. (2008). “Pitch strength decreases as F0 and harmonic resolution increase in complex tones composed exclusively of high harmonics.” J. Acoust. Soc. Am., 123, p.2670-9. [1] [2]
- Patterson, R.D., Handel, S., Yost, W.A. and Datta, A.J. (1996). “The relative strength of the tone and noise components in iterated rippled noise.” J. Acoust. Soc. Am., 100, p.3286-3294. [1] [2] [3] [4] [5] [6] [7] [8]
- Patterson, R.D., Yost, W.A., Handel, S. and Datta, A.J. (2000). “The perceptual tone/noise ratio of merged iterated rippled noises.” J. Acoust. Soc. Am., 107, p.1578-1588. [1]
- Yost, W.A. (1996). “Pitch of iterated rippled noise.” J. Acoust. Soc. Am., 100, p.511-518. [1]
- Yost, W.A., Patterson, R. and Sheft, S. (1996). “A time domain description for the pitch strength of iterated rippled noise.” J. Acoust. Soc. Am., 99, p.1066-1078. [1]
- Yost, W.A., Patterson, R. and Sheft, S. (1998). “The role of the envelope in processing iterated rippled noise.” J. Acoust. Soc. Am., 104, p.2349-2361. [1]